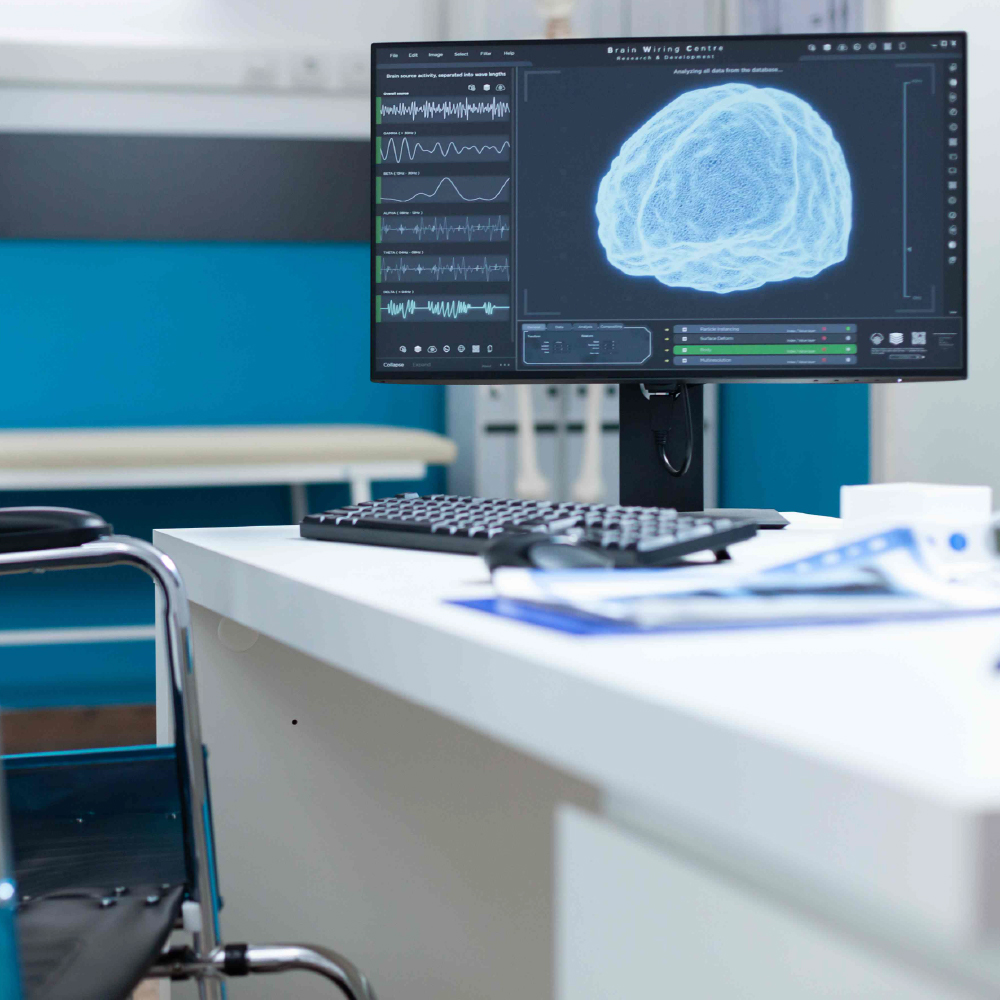
Radiology, the medical specialty that uses imaging to diagnose and treat diseases, has seen tremendous advancements over the past few decades. With the advent of artificial intelligence (AI), the future of radiology is set to undergo a significant transformation. AI’s ability to enhance imaging accuracy promises to revolutionize diagnostics, improve patient outcomes, and streamline radiology workflows. This article explores AI’s role in the future of radiology and how it is poise to enhance imaging accuracy.
The Evolution of Radiology and AI
Radiology has come a long way since Wilhelm Conrad Roentgen discovered X-rays in 1895. The development of techniques such as computed tomography (CT), magnetic resonance imaging (MRI), and ultrasound have revolutionized medical diagnostics. However, these advancements also brought challenges, including the need for highly trained specialists to interpret complex images and the potential for human error.
AI’s integration into medicine began with the development of algorithms capable of analyzing vast amounts of data. In radiology, AI has demonstrated its potential to assist in image interpretation, pattern recognition, and decision-making processes. Machine learning, a subset of AI, has been particularly instrumental in these advancements, enabling computers to learn from data and improve their performance over time.
Enhancing Imaging Accuracy with AI
One of the most promising applications of AI in radiology is automate image analysis. AI algorithms can quickly and accurately identify abnormalities in medical images, reducing the workload on radiologists and minimizing the risk of oversight. For example, AI can detect tiny lung nodules on chest X-rays or identify subtle brain anomalies on MRIs that might be miss by the human eye.
AI also excels in quantitative imaging, where it can measure and analyze specific features within an image. This capability is particularly valuable in oncology, where AI can assess tumor size, shape, and growth over time with high precision. Quantitative imaging provides clinicians with objective data that can guide treatment decisions and monitor disease progression.
Human interpretation of medical images can vary due to factors such as fatigue, experience, and cognitive biases. AI helps mitigate this variability by providing consistent and reproducible results. By standardizing image analysis, AI ensures that diagnoses are more reliable and less dependent on individual radiologists’ expertise.
AI-Powered Radiology Tools
Computer-aided detection (CAD) systems are among the earliest AI applications in radiology. These systems assist radiologists by highlighting suspicious areas in medical images that may warrant further investigation. CAD systems have proven particularly useful in mammography, where they help detect early signs of breast cancer.
Deep learning, a subset of machine learning, has shown remarkable success in image recognition tasks. In radiology, deep learning algorithms can be trained on large datasets of labeled images to recognize patterns indicative of specific diseases. For instance, deep learning models have demonstrated high accuracy in diagnosing pneumonia from chest X-rays and identifying diabetic retinopathy from retinal images.
Natural language processing (NLP) is another AI technology making waves in radiology. NLP algorithms can analyze radiology reports, extract relevant information, and identify patterns or trends. This capability enables radiologists to quickly access critical patient data and enhances the overall efficiency of the diagnostic process.
AI in Clinical Practice
Picture Archiving and Communication Systems (PACS) are integral to modern radiology departments, facilitating the storage and retrieval of medical images. Integrating AI into PACS allows for seamless incorporation of AI-powered tools into clinical workflows. Radiologists can access AI-generated insights directly from their PACS workstation, streamlining the diagnostic process.
AI can serve as a valuable clinical decision support tool, providing radiologists with evidence-based recommendations and highlighting potential diagnoses. This support is particularly beneficial in complex cases or when radiologists face a high volume of images to review. AI can act as a second pair of eyes, ensuring that no critical findings are overlooked.
AI is also transforming radiology education. AI-powered simulators and training platforms offer radiologists-in-training opportunities to practice interpreting images and receive feedback on their performance. These tools help accelerate the learning curve and ensure that new radiologists are well-prepared to handle the complexities of modern imaging.
Challenges and Ethical Considerations
The integration of AI in radiology raises important ethical and legal considerations. Ensuring the privacy and security of patient data is paramount. AI algorithms require large datasets for training, which can pose risks if not handled properly. Implementing robust data anonymization and encryption methods is essential to protect patient confidentiality.
AI algorithms are only as good as the data they are trained on. If training datasets are biased or lack diversity, AI models may produce skewed results. It is crucial to use representative datasets and continuously monitor AI systems for potential biases. Addressing these biases is essential to ensure equitable and accurate diagnostic outcomes for all patient populations.
The use of AI in medical practice requires regulatory approval to ensure safety and efficacy. Regulatory bodies such as the FDA in the United States have established guidelines for evaluating AI-based medical devices. Obtaining regulatory approval can be a lengthy and complex process, but it is necessary to ensure that AI tools meet rigorous standards.
The Future of AI in Radiology
AI’s ability to analyze vast amounts of data holds promise for personalized medicine. By integrating patient-specific data, such as genetic information and medical history, AI can help tailor diagnostic and treatment plans to individual patients. This approach has the potential to improve outcomes and reduce healthcare costs by targeting therapies more effectively.
The future of radiology will likely see the development of collaborative AI systems, where AI and radiologists work together in a symbiotic relationship. Rather than replacing radiologists, AI will augment their capabilities, allowing them to focus on complex cases and patient care. This collaboration will enhance the overall quality of radiology services and improve patient outcomes.
AI systems have the unique ability to continuously learn and improve from new data. As more medical images are analyzed and more cases are diagnosed, AI algorithms will become increasingly accurate and sophisticated. This continuous learning process ensures that AI remains at the forefront of medical innovation, driving ongoing advancements in radiology.
The future of radiology is undeniably intertwined with the advancements in artificial intelligence. AI’s role in enhancing imaging accuracy promises to revolutionize diagnostics, improve patient outcomes, and streamline radiology workflows. As AI continues to evolve, it is essential to address the challenges and ethical considerations associated with its use. By embracing AI’s potential and fostering collaboration between AI and radiologists, the future of radiology holds immense promise for a more accurate and efficient healthcare system.