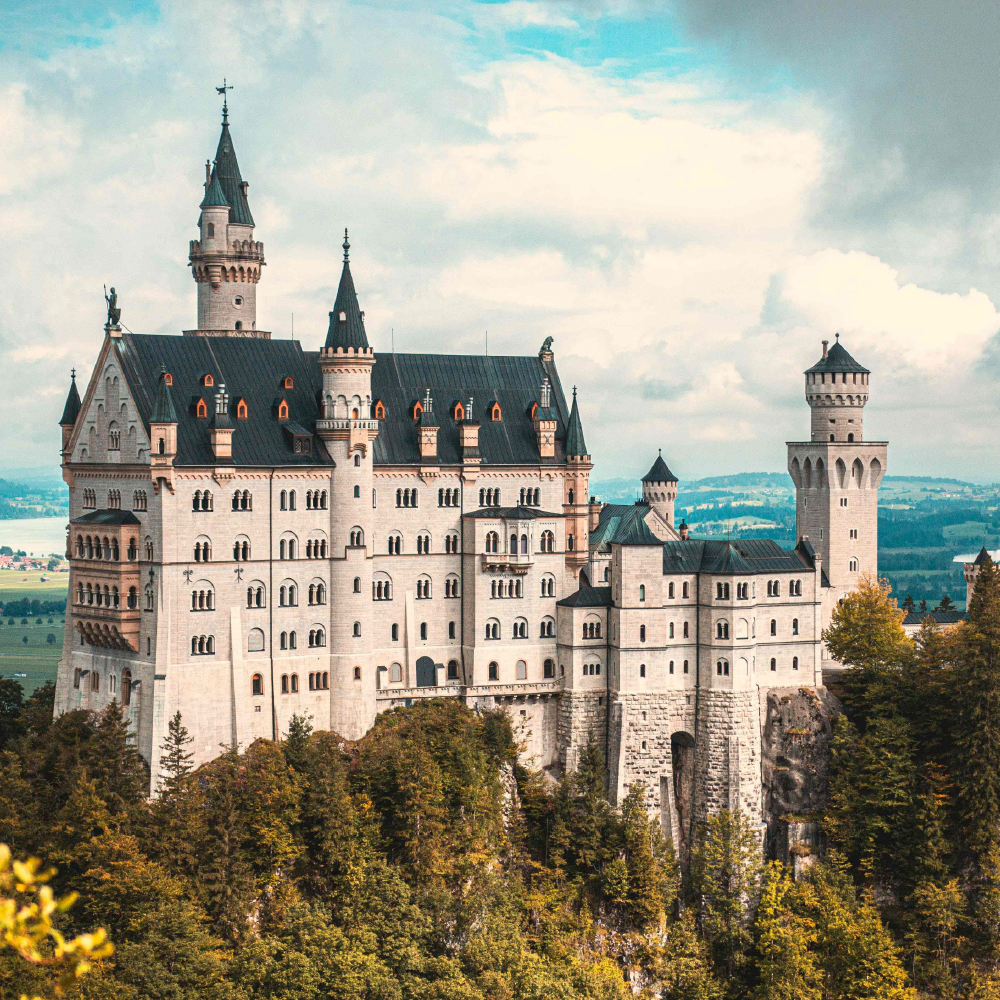
In the ever-evolving healthcare landscape, one field stands at the forefront of Revolutionizing radiology. As medical imaging technologies continue to advance, so does the need for accurate and timely diagnoses. In this digital age, the integration of artificial intelligence (AI) promises to revolutionize the practice of radiology, enhancing imaging accuracy, improving patient outcomes, and reshaping the future of healthcare delivery.
The Evolution of Medical Imaging: From Analog to Digital
The history of medical imaging is a tale of technological innovation and scientific discovery. From the discovery of X-rays by Wilhelm Conrad Roentgen in 1895 to the development of magnetic resonance imaging (MRI) and computed tomography (CT) scanning in the latter half of the 20th century, medical imaging has revolutionized how physicians diagnose and treat disease.
In recent decades, the transition from analog to digital imaging has further accelerated the pace of innovation in radiology. Digital imaging technologies, such as digital radiography (DR) and picture archiving and communication systems (PACS), have streamlined capturing, storing, and sharing of medical images, improving efficiency and enabling more comprehensive patient care.
The Promise of Artificial Intelligence in Radiology
Artificial intelligence, particularly in the form of machine learning and deep learning algorithms, holds immense promise for the field of radiology. By analyzing vast amounts of imaging data with unprecedented speed and accuracy, AI has the potential to assist radiologists in detecting abnormalities, predicting disease progression, and personalizing treatment plans.
One of the most compelling applications of AI in radiology is computer-aided diagnosis (CAD), where algorithms are trained to detect patterns and anomalies in medical images. These AI-powered systems can help radiologists identify subtle signs of disease that may be overlooked by the human eye, leading to earlier and more accurate diagnoses.
Additionally, AI algorithms can analyze longitudinal imaging data to track disease progression over time, providing valuable insights into the effectiveness of treatment strategies and guiding clinical decision-making. In oncology, AI-powered imaging tools assess tumor response to therapy, predict patient outcomes, and optimize treatment protocols.
Enhancing Imaging Accuracy Through AI
Integrating AI into the radiology workflow can significantly enhance imaging accuracy and improve patient outcomes across a wide range of medical specialties. In diagnostic imaging, AI algorithms can assist radiologists in detecting and characterizing abnormalities, reducing the risk of false positives and negatives and improving diagnostic confidence.
For example, in detecting breast cancer, AI-powered mammography systems have demonstrated the ability to identify subtle signs of malignancy with high sensitivity and specificity, potentially leading to earlier detection and more effective treatment. Similarly, in neuroimaging, AI algorithms can aid in detecting intracranial hemorrhage, ischemic stroke, and other neurological conditions, enabling timely intervention and improved patient outcomes.
In addition to improving diagnostic accuracy, AI can also optimize the efficiency of radiology workflows, allowing radiologists to focus their time and expertise on complex cases requiring clinical judgment. By automating routine tasks such as image interpretation and report generation, AI-powered systems can help reduce interpretation times, minimize errors, and increase productivity, ultimately enhancing the delivery of patient care.
Challenges and Considerations in AI Implementation
Despite its immense potential, the widespread adoption of AI in radiology has challenges and considerations. Chief among these is the need for robust validation and regulatory approval of AI algorithms to ensure their safety, efficacy, and clinical utility. Additionally, concerns regarding data privacy, security, and interoperability must be addressed to facilitate the seamless integration of AI into existing radiology workflows.
Moreover, the successful implementation of AI in radiology requires collaboration and partnership between radiologists, data scientists, engineers, and other stakeholders. Interdisciplinary teams must work together to develop and deploy AI algorithms tailored to the specific needs and challenges of the radiology practice, considering factors such as patient demographics, disease prevalence, and imaging protocols.
The Future of Radiology: Embracing AI as a Catalyst for Change
As we look to the future of radiology, it is clear that artificial intelligence will play an increasingly prominent role in enhancing imaging accuracy, improving diagnostic efficiency, and transforming patient care. By harnessing the power of AI to analyze and interpret medical images with unprecedented speed and accuracy, radiologists can unlock new insights, identify subtle signs of disease, and deliver more personalized and precise treatment strategies.
In this era of rapid technological advancement, integrating AI into the radiology workflow can revolutionize medicine, reshaping how we diagnose, treat, and manage disease. As we navigate this transformative journey, it is essential to embrace innovation, foster collaboration, and uphold the highest standards of patient care, ensuring that AI catalyzes positive change in radiology and beyond.